School of Geographical Science and Planning, Sun Yat-Sen University / University of Cincinnati, Ohio, USA
"Remote Sensing" impact factor: 3.244
Mangrove forest is a valuable resource. Mangrove ecosystem is one of the four most productive ecosystems in the world. It has great ecological and economic value. Remote sensing technology has large coverage area, short data update period and high spatial resolution. Its characteristics have become one of the main technologies for mangrove monitoring at home and abroad.
Taking S185 airborne hyperspectral data as an example, CART and CFS are used to select the characteristic wavelengths, and the object-oriented KNN and SVM classification algorithms are used to classify the mangrove species in the Qi'ao Island Natural Scenic Area of ​​Zhuhai City, Guangdong Province. The accuracy reached 76.12% (Kappa = 0.73) and 82.39% (Kappa = 0.801) respectively. By classifying the elevation information obtained by DSM data with S185 airborne hyperspectral data, the classification accuracy of KNN and SVM was increased to 82.09. %(Kappa = 0.797) and 88.66% (Kappa = 0.871). The results show that the airborne hyperspectral data based on S185 can be used for the classification of mangroves, and it also provides a reference value for multi-source data processing methods based on hyperspectral imagery.
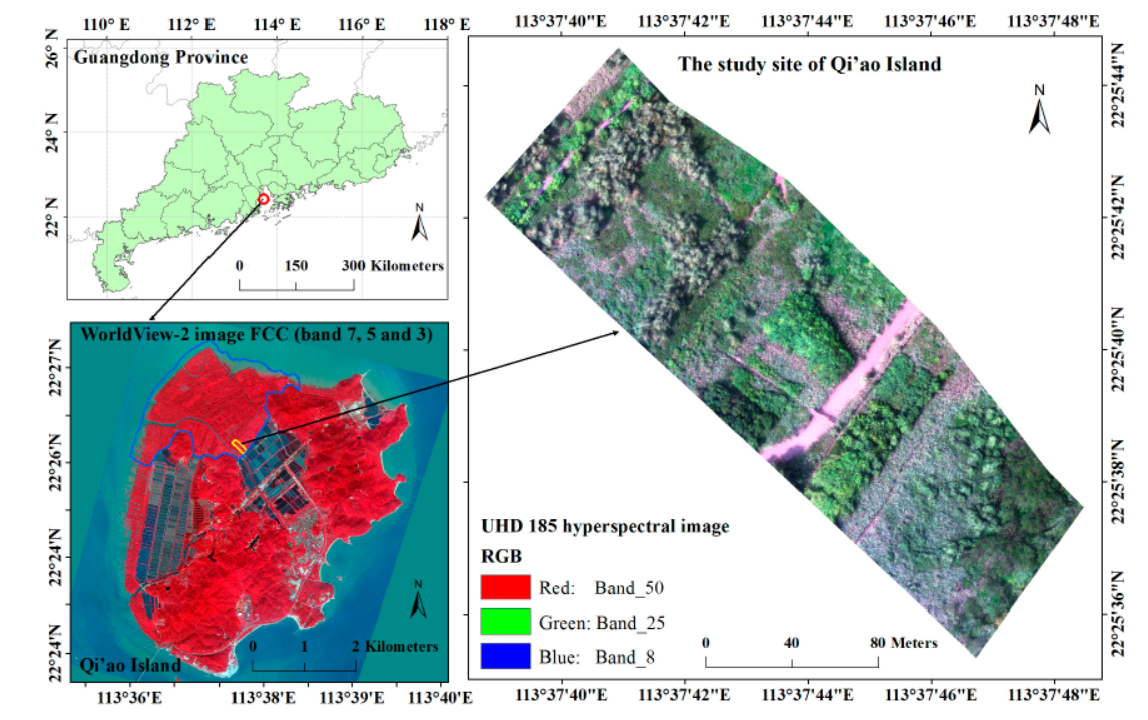
The left side of Figure 1 is the experimental study area, and the right side is the RGB composite image of the S185 airborne hyperspectral imager data.
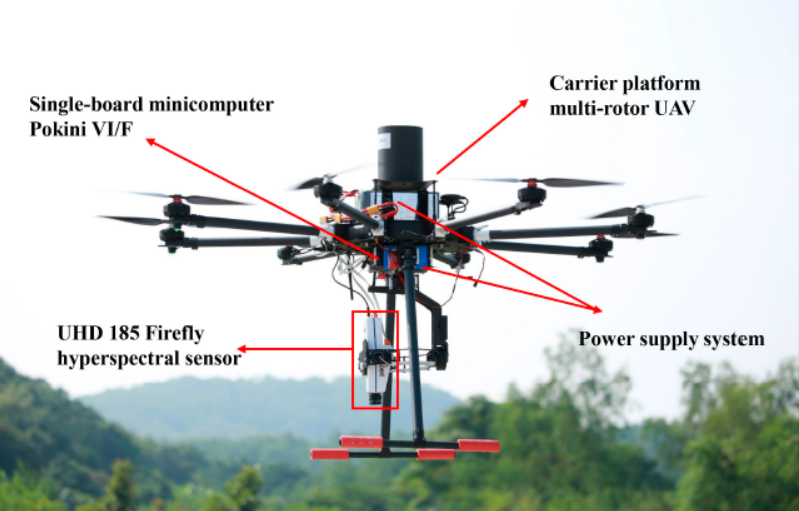
Figure 2 UAV equipped with S185 airborne high-speed imaging spectrometer
Table 1 Selection and division of mangrove ground training set and verification set
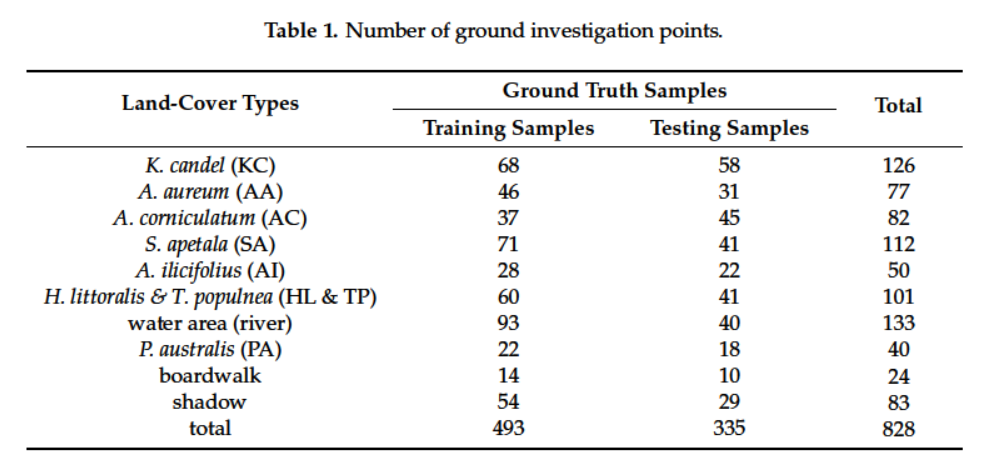
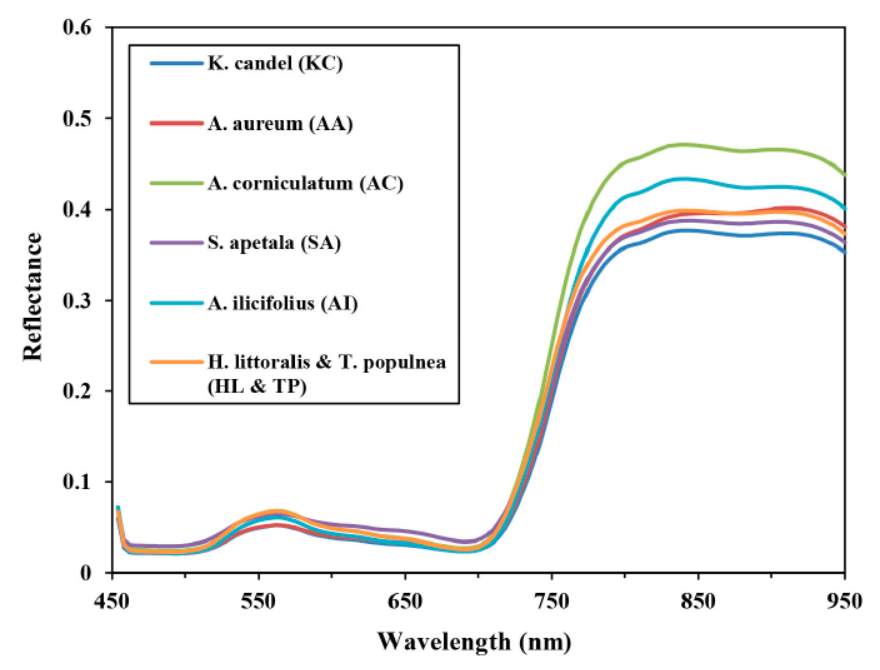
Figure 3 S185 reflectance spectral data of different mangrove species
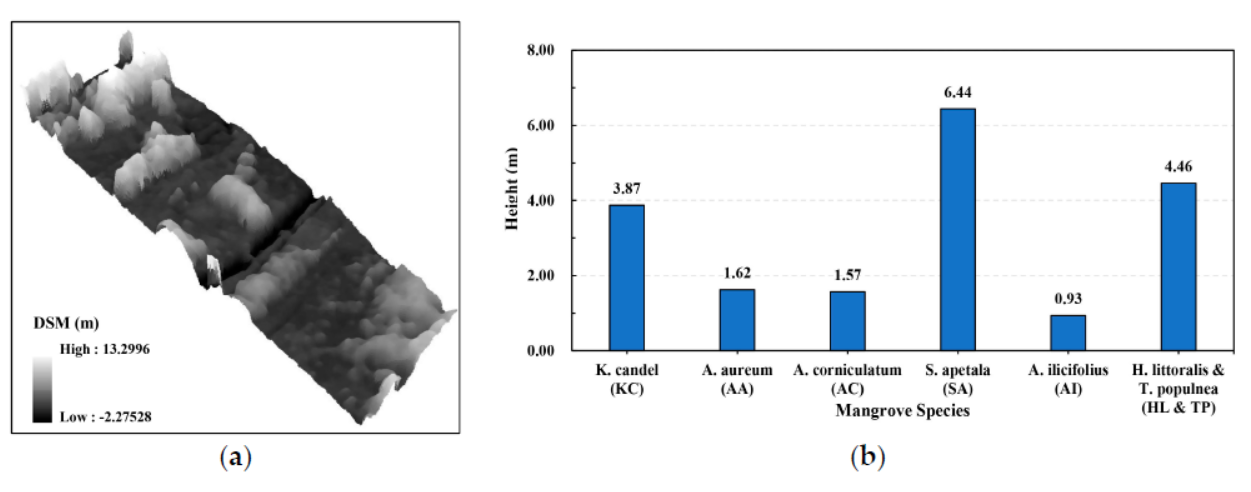
Figure 4 (a) shows the 3D display of the study area DSM, and (b) shows the average height information of different tree species.
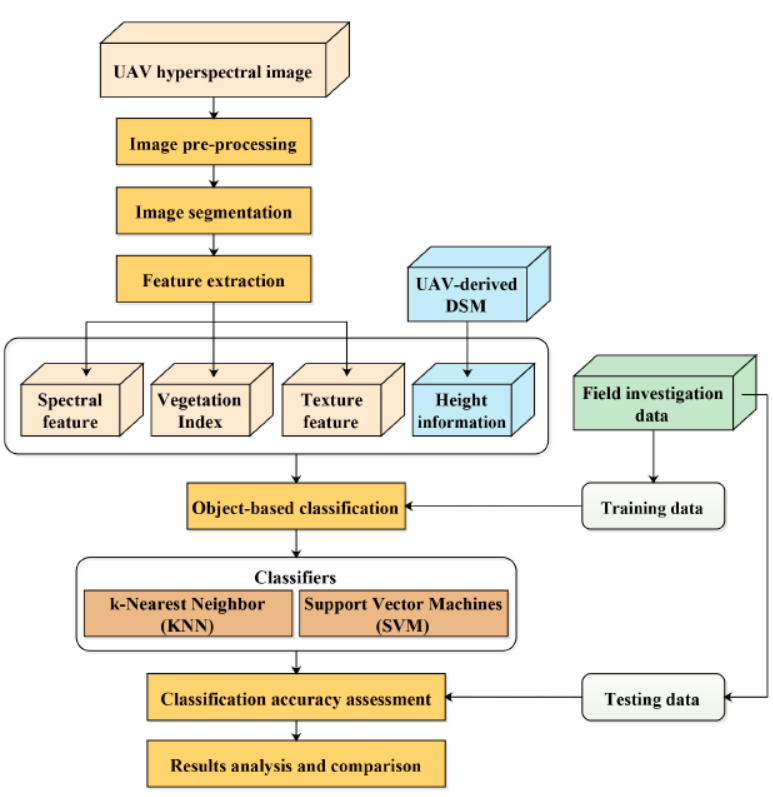
Fig.5 Flow chart of mangrove object-oriented classification based on S185 airborne hyperspectral data
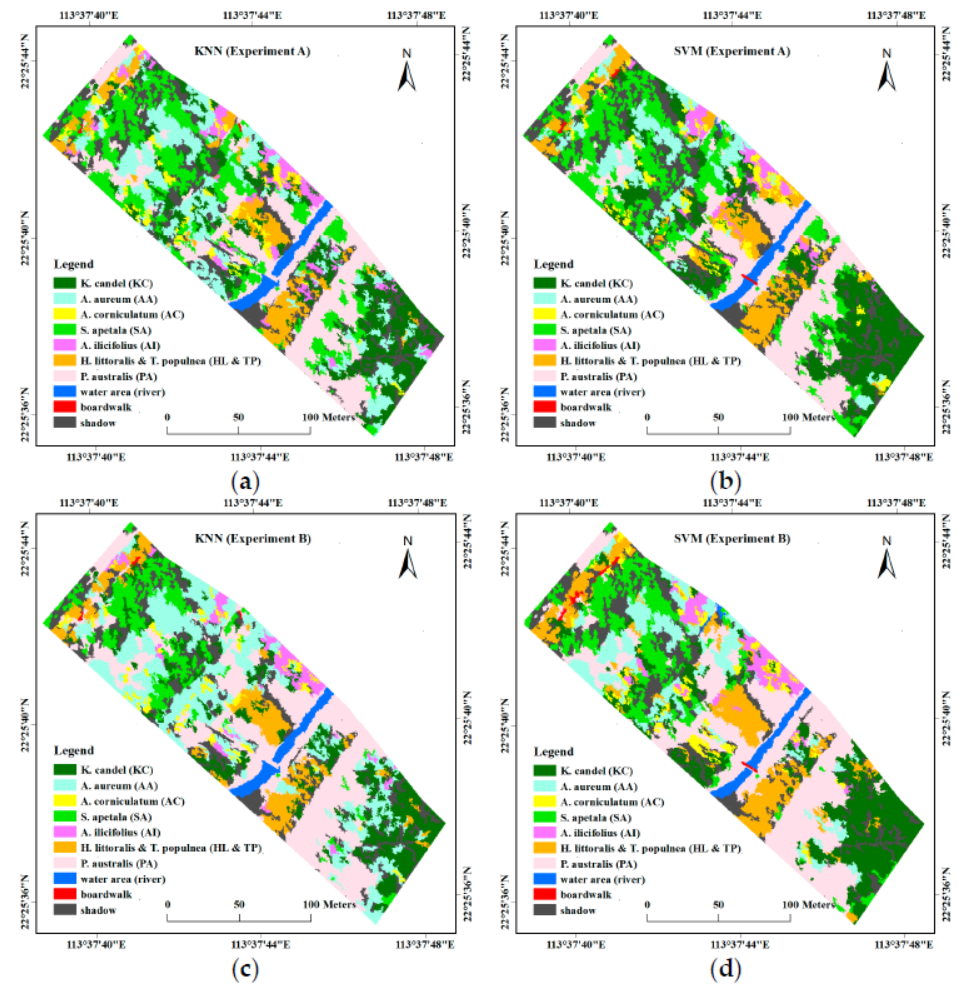
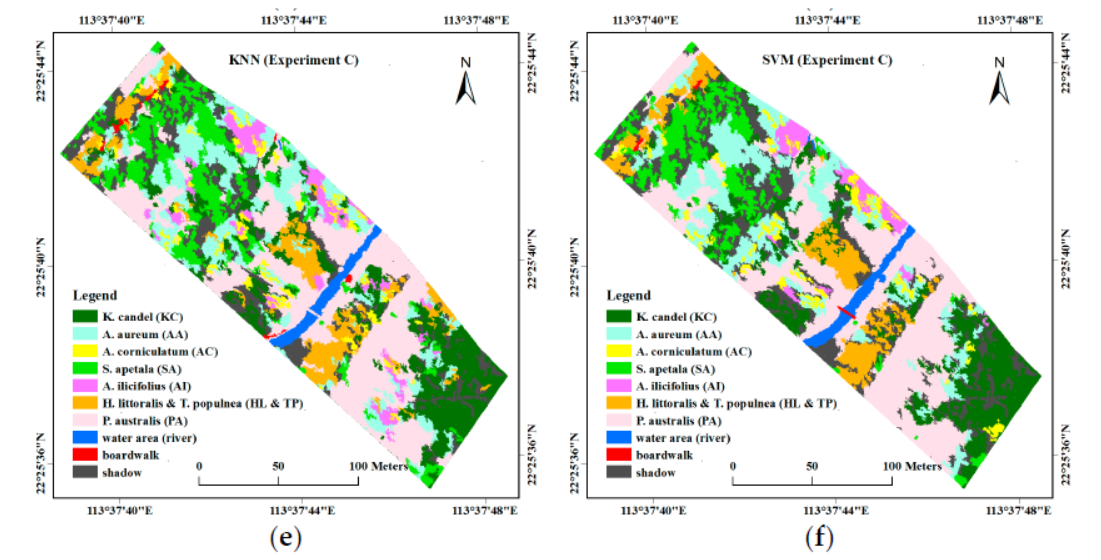
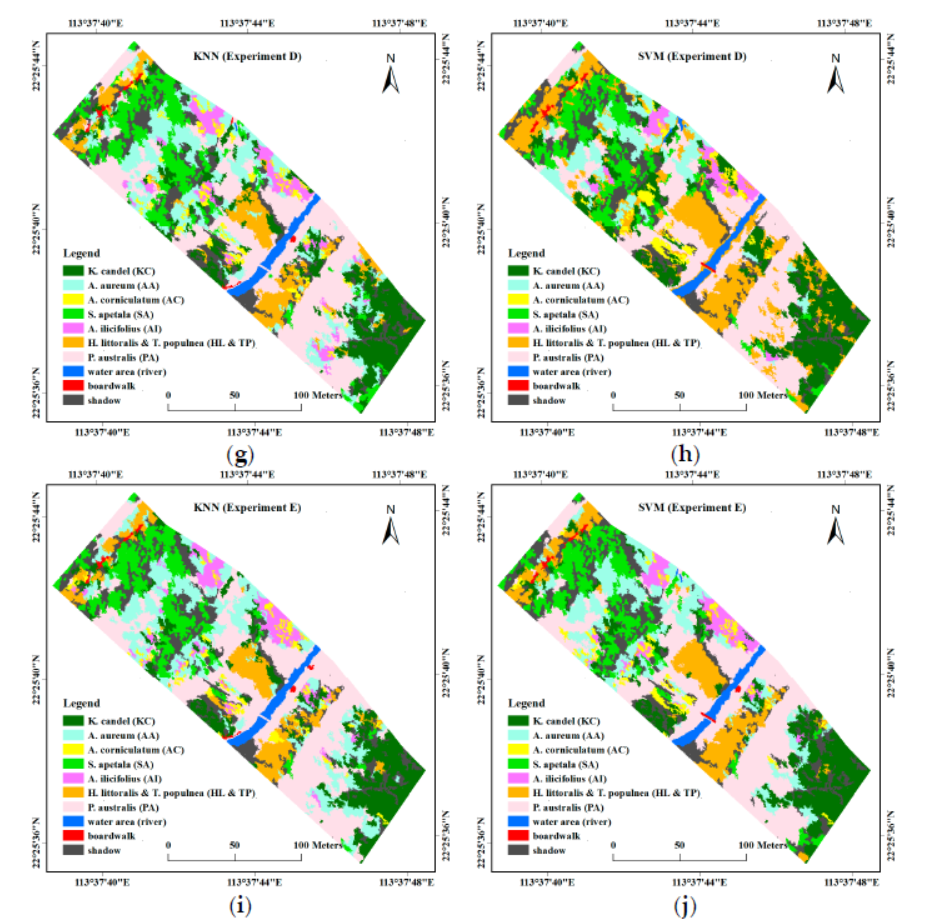
Figure 6. Image classification results based on different classification parameters of different feature parameters
(a) (b): KNN and SVM classification results based on 32 feature bands selected by CART
(c) (d): KNN and SVM classification results based on 32 feature bands and vegetation indices and texture features selected by CART
(e) (f): KNN and SVM classification results based on 32 feature bands and elevation information selected by CART
(g) (h): KNN and SVM classification results based on 32 feature bands and vegetation indices, texture features, and elevation information selected by CART
(i) (j): 14 characteristic parameters selected based on CFS (band 10, band 23, band 62 and band 91, four hyperspectral VIs, ie, NDVI, TCARI, MCARI2, PRI, five textural features, ASM (band 50 ), COR (band 8 and 25), MEAN (band 8), and StdDev (band 8), and UAV-derived DSM)
Table 2 Classification results of KNN classification algorithms based on different characteristic parameters
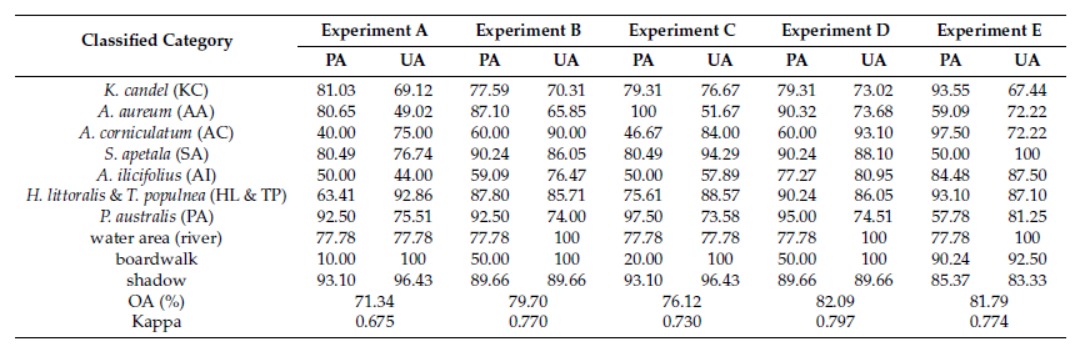
Table 3 Classification results of SVM classification algorithms based on different characteristic parameters
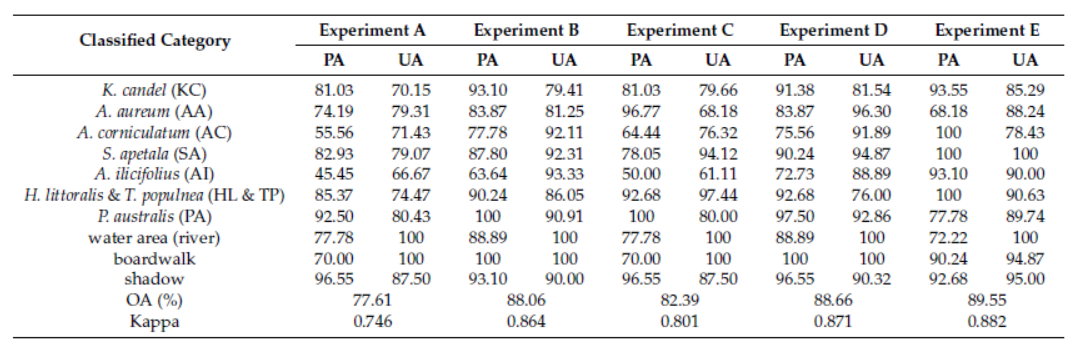
in conclusion:
1 Comparing the classification accuracy of KNN and SVM, the algorithm of SVM is better than KNN, and its combination with the elevation data of the forest makes the classification accuracy as high as 88.66% (Kappa = 0.871);
2 Data dimensionality reduction can improve the classification accuracy and classification ability of the SVM algorithm;
3 Combine high-precision spectral information (including vegetation index) with high-precision spatial information (including texture features and elevation information) to obtain high-precision mangrove classification results, especially the elevation information of forests can effectively solve the same spectrum. The classification problem of foreign bodies and foreign bodies with the same spectrum.
Original link: http://
DongGuan Lucky Pet Products Co., Ltd. , https://www.dgpetproducts.com